About
I am a researcher working at NTT Communication Science Laboratories (research institute in the Japanese telephone company, named NTT). I received a Ph.D. in informatics from Kyoto University under the supervision of Hisashi Kashima. Prior to joining NTT, I was majoring in systems biology and bioinformatics at Keio University and the University of Tokyo, supervised by Akira Funahashi and Satoru Miyano.
News
2024.04
Our paper entitled Differentiable Pareto-Smoothed Weighting for High-Dimensional Heterogeneous Treatment Effect Estimation has been accepted to UAI2024 (Acceptance rate 27.0%)! We propose differentiable Pareto smoothing, an end-to-end IPW weight correction framework for CATE estimation.
2024.04
Joint work entitled Meta-learning for heterogeneous treatment effect estimation with closed-form solvers has been accepted to Machine Learning (Impact Factor: 7.5)! We tackled the few-shot CATE estimation problem where we have access to only a few observational data instances.
2023.12
Joint work entitled Uncertainty Quantification in Heterogeneous Treatment Effect Estimation with Gaussian-Process-Based Partially Linear Model has been accepted to AAAI2024! We have developed a Bayesian semi-parametric model for uncertainty quantification in CATE estimation.
2023.09
My grant proposal entitled Causal Inference from Incomplete Data for Fair Machine Learning Predictions has been accepted to JST (ACT-X; Acceptance rate 19.9%)! I will tackle the difficulties in causal discovery, treatment effect estimation, and causality-based fairness.
2022.09
I have got Ph.D. (Informatics) from Kyoto University. The dissertation title is Causal Inference for Scientific Discoveries and Fairness-Aware Machine Learning (PDF).
2022.05
Our paper entitled Feature Selection for Discovering Distributional Treatment Effect Modifiers has been accepted to UAI2022! We consider the concept of distributional treatment effect modifiers to correctly understand the causal mechanisms of treatment effect heterogeneity.
Biography
I am working at the intersection between causal inference and machine learning. My current research aims at developing fundamental techniques for causal inference from incomplete data, i.e., real-world data with several difficulties, including, but not limited to small sample size, high dimensionality, and complex measurement noise. I believe that such causal inference techniques offer an essential foundation for making scientific knowledge discoveries and achieving reliable machine learning.
Education
Ph.D. of Informatics
2019.10 - 2022.09
Kashima Lab., Dept. of Intelligence Science & Technology, Graduate School of Informatics, Kyoto University, Japan.
Ph.D. Dissertation: Causal Inference for Scientific Discoveries and Fairness-Aware Machine Learning
Key Words: Causal discovery, Treatment effect estimation, Machine learning and fairness
Master of Information Science & Technology
2013.04 - 2015.03
Miyano Lab., Dept. of Computer Science, Graduate School of Information Science and Technology, The University of Tokyo, Japan.
Master Thesis: An Infinite Relational Model for Integrative Analysis of Cancer Genome Data
Key Words: Bioinformatics, Omics data analysis, Non-parametric Bayesian models, Survival time analysis
Bachelor of Science
2009.04 - 2013.03
Funahashi Lab., Dept. of Biosciences and Informatics, Faculty of Science and Technology, Keio University, Japan.
Bachelor Thesis: Developing Biochemical Network Simulator with Adaptive Step Size Numerical Integration
Key Words: Systems biology, Ordinary differential equations, Numerical integration, Bifurcation analysis
Professional Experience
Principal investigator
2023.10 - 2026.03
ACT-X, Japan Science and Technology Agency (JST)
- Grant proposal: Causal Inference from Incomplete Data for Fair Machine Learning Predictions
- 4,500,000 JPY (+ 500,000 JPY)
- Acceptance rate: 19.9%
Research scientist
2015.04 - Present
Learning and Intelligent Systems Group, Innovative Communication Laboratory, Communication Science Laboratories, Kyoto, Japan.
- Causal discovery
- Granger causality inference via supervised learning (IJCAI2018, TOM2018)
- Treatment effect estimation
- Selection of distributional treatment effect modifiers for causal mechanism understanding (UAI2022)
- Uncertainty quantification of conditional average treatment effect (CATE) via Gaussian-process-based partially linear model (AAAI2024)
- Weighted representation learning with differentiable Pareto-smoothed weights for CATE estimation from high-dimensional observational data (UAI2024)
- CATE estimation under few-shot setting via meta-learning of meta-learner models (Machine Learning 2024)
- Machine learning and causality-based fairness
- Achieving path-specific counterfactual fairness under milder assumptions (AISTATS2021, DAMI2022)
Selected Research Topics
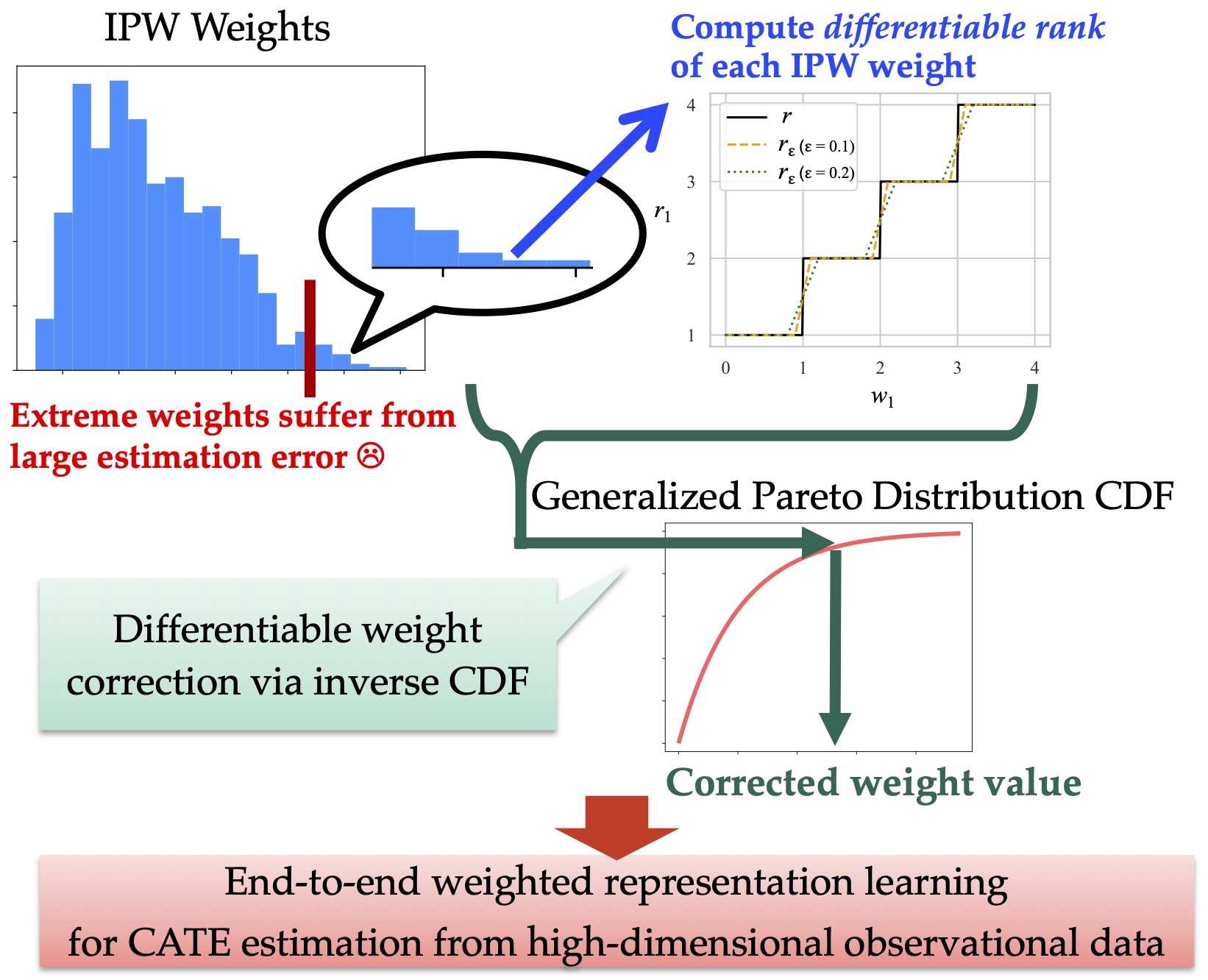
Differentiable Pareto-Smoothed Weighting for High-Dimensional Heterogeneous Treatment Effect Estimation
Assessing the effects of a treatment (e.g., drug administration) offers a deep understanding of treatment effect heterogeneity across individuals and is helpful for effective decision-making in various fields, such as precision medicine, personalized education, and targeted advertisement.
To estimate heterogeneous treatment effects from observational data, one needs to distinguish causal effects from so-called spurious correlation, which is induced by confounders, i.e., the features of an individual that influence their treatment choices and outcomes. To make such a distinction, practitioners attempt to add as many features as possible to their dataset because it is often unclear which features correspond to confounders. As a result, they must face the challenge of high-dimensional heterogeneous treatment effect estimation.
A promising approach for high-dimensional setups is weighted representation learning, which decomposes observed features into the representations of confounders and other features by minimizing the weighted prediction loss. Such data-driven feature decomposition is helpful to avoid losing predictive information of adjustment variables, which are not confounders but are predictive of potential outcomes. In practice, however, this approach suffers from performance degradation due to the numerical instability of the weight values, which are given by the inverse of conditional probabilities based on a technique called inverse probability weighting (IPW).
To overcome this issue, we propose an effective weight correction framework that can be used in an end-to-end fashion. To achieve this goal, we combine Pareto smoothing in extreme value statistics and differentiable ranking in machine learning. The resulting differentiable Pareto-smoothed weighting framework allows us to effectively learn the feature representations from high-dimensional data and to achieve high treatment effect estimation performance.
-
Yoichi Chikahara, Kansei Ushiyama. Differentiable Pareto-Smoothed Weighting for High-Dimensional Heterogeneous Treatment Effect Estimation. Proc. of the 40th International Conference on Uncertainty in Artificial Intelligence. Barcelona, Spain, July 2024 (UAI2024; Acceptance Rate: 27%) [Preprint] [Openreview] [Proceedings] [Paper(PDF)] [Poster(PDF)] [Code]
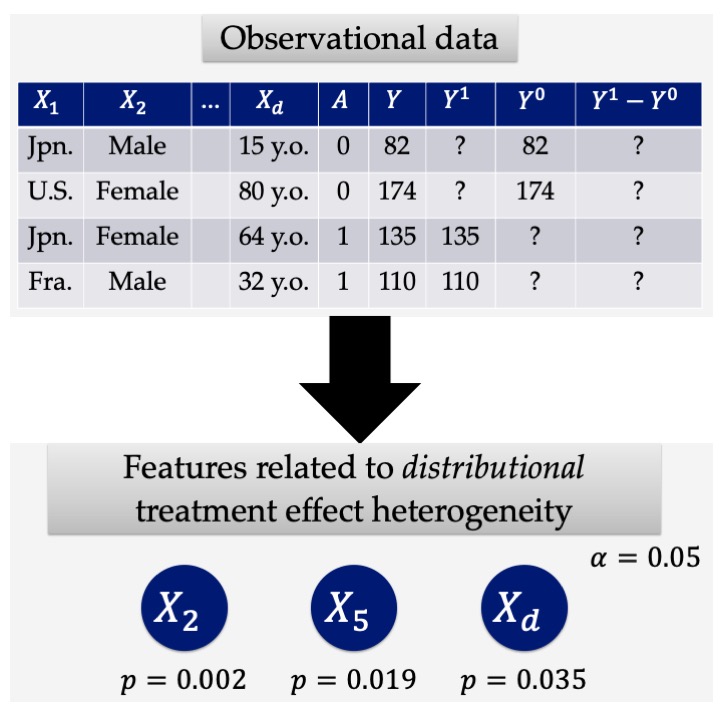
Feature Selection for Discovering Distributional Treatment Effect Modifiers
The statistical estimation of the effects of a treatment (or an intervention) is crucially important in various applications, such as precision medicine, personalized education, and targeted advertisement. For instance, predicting the effects of a medical treatment (e.g., drug administration and vaccination) on health status is essential to improve precision medicine, and inferring the effects of education and training programs is helpful for personalized education.
The degrees of such treatment effects often vary depending on individuals; if so, elucidating why such treatment effect heterogeneity exists is a topic of great importance. A popular traditional approach to explaining treatment effect heterogeneity is to select the feature attributes of an individual that are relevant to the degree of a treatment effect. The difficulty of this feature selection problem is that we cannot measure a treatment effect for each individual because it is defined as a difference between the potential outcomes, i.e., the outcomes when an individual is treated and not treated, which are never jointly observed. For this reason, the existing methods use an average treatment effect across individuals with an identical attribute, which can be estimated from the observed data. However, such a mean-based methods may overlook important features if they do not affect the average treatment effect but do influence other distribution parameters, such as treatment effect variance.
To overcome this weakness of the existing methods, we propose a feature selection framework for discovering distributional treatment effect modifiers. To establish such a framework, we develop a feature importance measure based on the kernel maximum mean discrepacy (MMD) and derive a multiple-testing-based algorithm that can control the type I error rate (i.e., the proportion of false-positive results) to the desired level.
-
Yoichi Chikahara, Makoto Yamada, Hisashi Kashima. Feature Selection for Discovering Distributional Treatment Effect Modifiers. Proc. of the 38th International Conference on Uncertainty in Artificial Intelligence. Eindhoven, Netherlands, August 2022 (UAI2022) [Preprint] [Openreview] [Proceedings] [Paper(PDF)] [Spotlight Slides(PDF)] [Poster(PDF)]
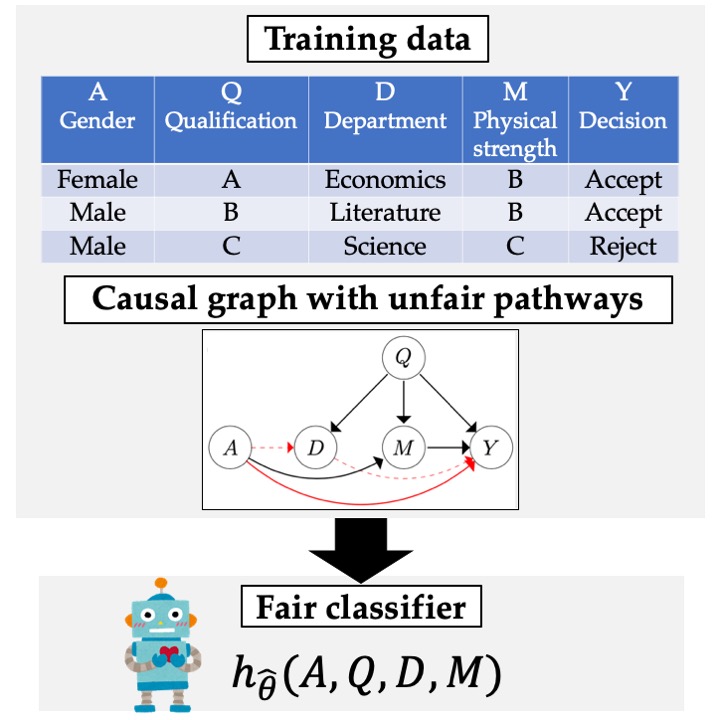
Learning Individually Fair Classifier with Path-Specific Causal-Effect Constraint
Machine learning is increasingly being used to make decisions that severely affect people's lives (e.g., hiring, lending, and recidividsm prediction). To achieve these applications, it is crucially important to learn a fair predictive model for making decisions that are fair with respect to a sensitive feature (e.g., gender, race, religion, disabilities, and sexual orientation). It is difficult to judge whether or not the decision is discriminatory because what we feel discriminatory often depends on each real-world scenario. For instance, when making hiring decisions for applicants for physically-demanding jobs, it might not be discriminatory to reject applicants due to physical strength.
Such a prior knowledge on what decisions should be regarded as discriminatory can be depicted as a causal graph with unfair pathways. Several existing methods have been proposed that learn fair predictive models by imposing a constraint on the causal effects along those unfair pathways; however, none of them cannot guarantee fairness for each individual without making impractical assumptions on the data.
To resolve this issue, we consider an optimization problem where the unfair causal effects are controlled to be zero for each individual. To do so, we define Probability of Individual Unfairness (PIU), which is the probability that the causal effect for an individual is not zero, and solve an optimization problem that constrains PIU to zero. Although this is difficult since PIU cannot be estimated from the data, we derive an upper bound on PIU via the notion of correlation gap and propose to solve an optimization problem that constrains the upper bound to zero.
-
Yoichi Chikahara, Shinsaku Sakaue, Akinori Fujino, Hisashi Kashima. Learning Individually Fair Classifier with Path-Specific Causal-Effect Constraint. Proc. of the 24th International Conference on Artificial Intelligence and Statistics. Online, April 2021 (AISTATS2021) [Preprint][Proceedings] [Paper (PDF)] [3-min. Video (Link)] [Slides (PDF)] [Poster (PDF)]
-
Yoichi Chikahara, Shinsaku Sakaue, Akinori Fujino, Hisashi Kashima. Making Individually Fair Predictions with Causal Pathways. Special Issue on "Bias and Fairness in AI", Data Mining and Knowledge Discovery (DAMI), 2022 [Article] [View-only shared link]
-
"Accurate and Fair Machine Learning based on Causality", The 6th StatsML Symposium (StatsML2022), Online, February 2022. [Abst] [Slides]
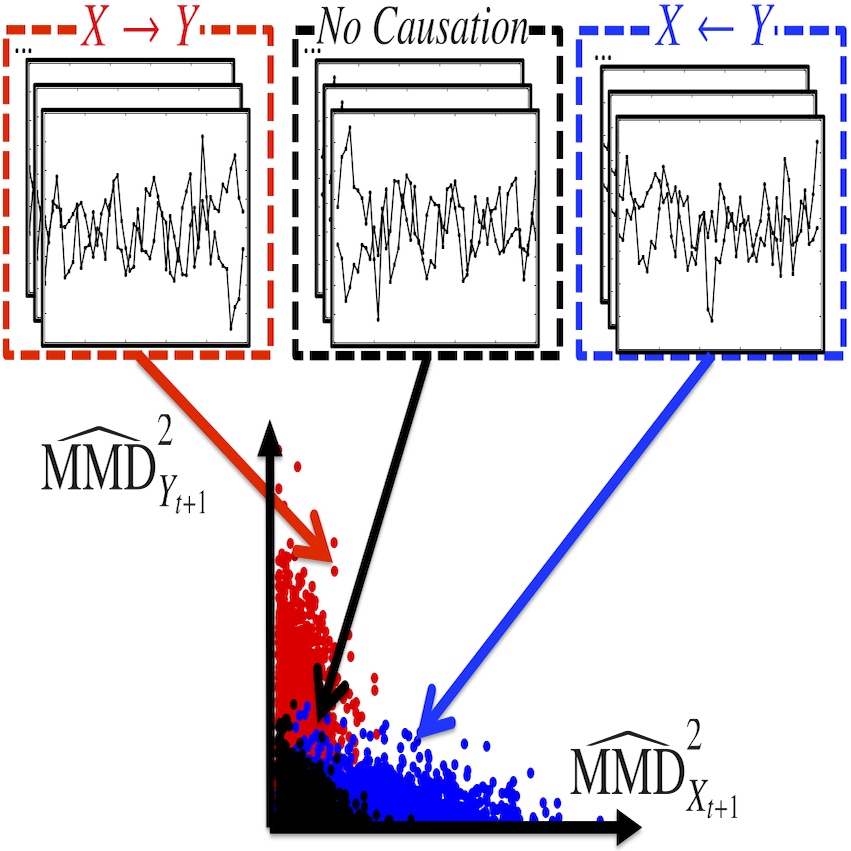
Causal Inference in Time Series via Supervised Learning
Discovering causal relationships in time series is one of the most important tasks in time series analysis and has key applications in various fields. For instance, finding the causal relationship indicating that the research and development (R&D) expenditure X influences the total sales Y, but not vice versa, (i.e., X->Y) will be a help for decision making in companies. In addition, discovering causal (regulatory) relationships between genes from time series gene expression data is one of the central tasks in bioinformatics.
For these applications, the metric of the temporal causality called Granger causality [Granger 1969] has been widely used. The definition is very simple: if the past values of X are "helpful" in predicting the future values of Y, then X is the cause of Y. To evaluate the "helpfulness" in prediction, many traditional methods use regression models, which are the mathmatical expressions representing the relationships between variables. When we use an appropriate regression model that can be well fitted to the data, we can identify correct causal directions with these methods. However, it is not easy to select an appropriate regression model for each data because it requires a deep understanding of the data (e.g., we need to consider the amount of data, the relationship between variables, and the noise in data).
Our goal is to build a novel approach that does not require a deep understanding of the data. To do so, we propose a supervised learning approach that utilizes a classifier instead of regression models. Specifically, we infer causal relationships by training a classifier that assigns ternary causal labels (X->Y,X<-Y, No Causation) to time series.
-
Yoichi Chikahara, Akinori Fujino. Causal Inference in Time Series via Supervised Learning. Proc. of the 27th International Joint Conference on Artificial Intelligence, Stockholm, Sweden, July 2018 (IJCAI2018; Acceptance Rate: 20%)[Proceedings] [Paper] [Slides] [Poster]
-
"Causal Inference in Time Series via Supervised Learning", Top Conference Session (Machine Learning) Forum on Information Technology (FIT2019), Okayama University, Okayama, September 2019 [PDF]
Publications
See here for my publication list.
Skills
I am into foreign languages. I have passed the pre-1st grade of Diplome d'Aptitude Pratique au Francais (i.e., Test in Practical French Proficiency), whose acceptance rate was 20.0% (in 2023).
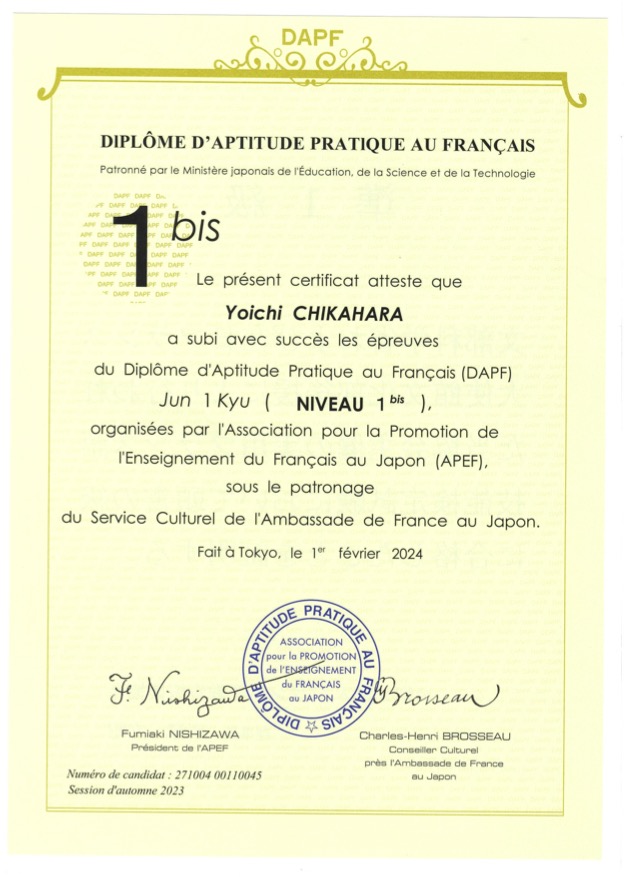
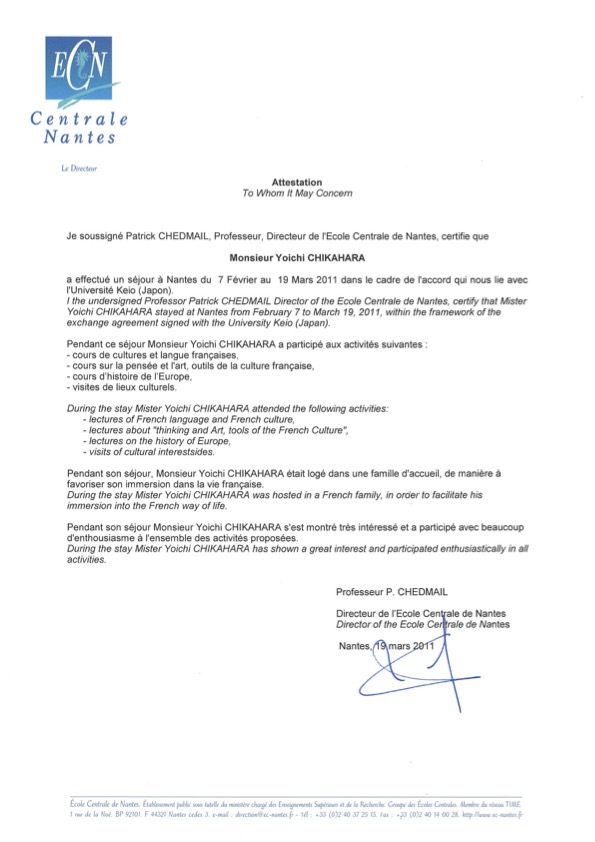
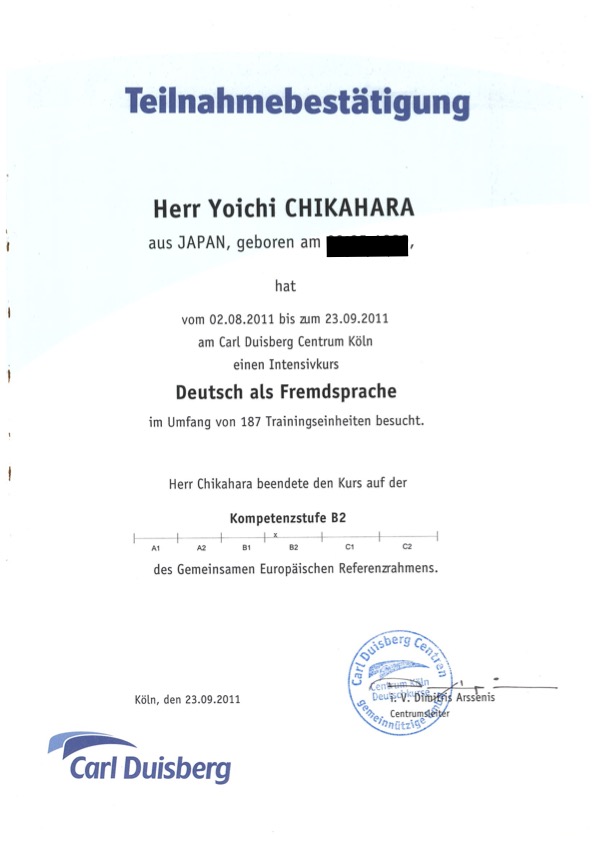
Contact
Please feel free to ask any questions or inquiries. We welcome research collaboration proposals, invitations for talks, and other inquiries related to our reserach activities.
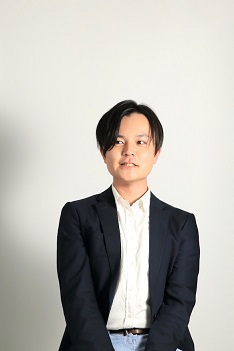
Location:
2-4 Hikaridai, Seika-cho, Soraku-gun, Kyoto, 619-0237, Japan
Email:
chikahara.yoichi (ζ) gmail.com